AI Helps Uncover Long-Term Effects of Antidepressants
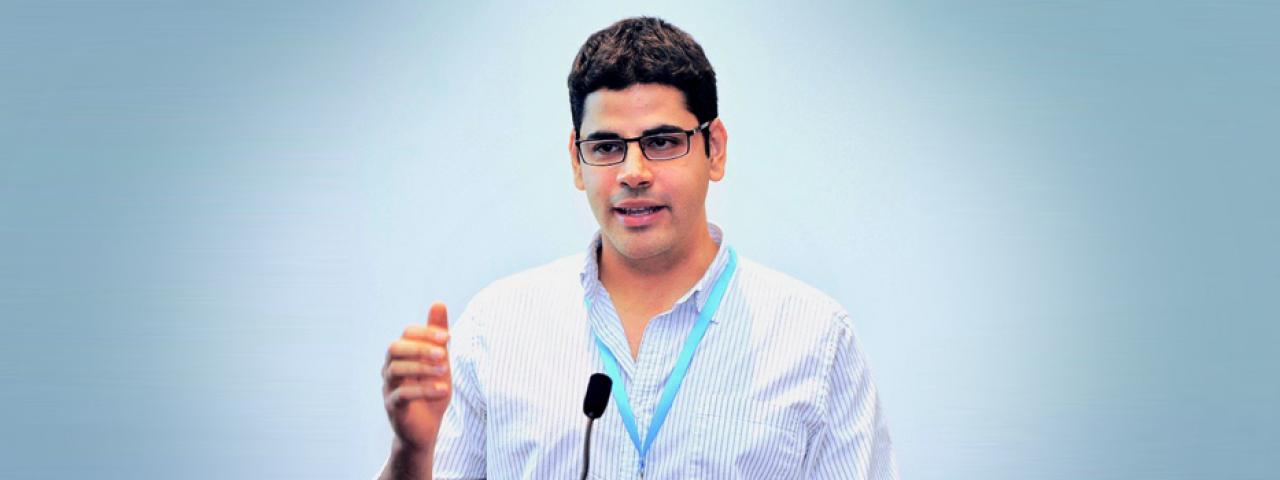
When NYU Shanghai Assistant Professor of Information Systems and Business Analytics Bruno Abrahao began studying artificial intelligence (AI), he never envisioned that his work might shape the world of psychiatric medicine.
That changed after Abrahao served as a teaching assistant at a top research university in the United States. He discovered that many of his students’ academic issues were rooted in something deeper: depression and anxiety.
“I had students reaching out to me, saying, ‘I’m very depressed, I can’t study, I can’t concentrate,’ or even worse, ‘I took out a loan to come here, and I’m not getting good grades. I can’t go back to my country with these grades, so I'm really anxious and I don’t know what to do.’”
Although Abrahao helped the students get the psychiatric treatment they needed, he often felt frustrated by the mixed results of the medications students received.
“Sometimes they got worse when they started taking medication,” said Abrahao. “So I became interested in using AI to illuminate this issue.”
Abrahao and fellow researchers from Georgia Tech, Microsoft Research, and Harvard Medical School have developed an AI-based method to enable mental health practitioners to understand the long-term effects of psychiatric medications, as well as their effects across populations that are too big and too varied for clinical trials to evaluate. Their research was published in the June 14 issue of the Proceedings of the Association for the Advancement of Artificial Intelligence, and won the Outstanding Paper Award at the International AAAI Conference on Web and Social Media in Munich, Germany.
Adapting a machine learning model originally developed for Reddit posts, the team pinpointed over 23,000 Twitter users whose posts indicated that they were taking any one of 49 popular psychiatric medications and estimated when they began and ended their treatment.
Next, the model found a control group of 280,000 Twitterers who used depressive language and who had similar social media habits, but who were not taking psychiatric medications. Using natural language processing techniques and observations of users’ posting behaviors, the researchers analyzed changes in mood, cognition, and thoughts of suicide in both groups in order to track the effects of psychiatric treatment.
The results are striking: posts by Twitter users who took a family of drugs called SSRIs - which includes many of the most popular antidepressants - showed long-term worsening of symptoms including anxiety, depression, psychosis, and suicidal ideation. In contrast, the posts of users who took an older group of drugs called TCAs showed more improvement in depressive symptoms over the course of the study’s two-year term.
Although psychiatrists and patients have expressed doubts about the effectiveness of SSRIs for years, Abrahao and his colleagues argue that their research substantiates the severity and scale of these negative effects in a large population.
Ultimately, the team’s research provides convincing evidence that psychiatrists, as well as the pharmaceutical industry, can look to social media to evaluate patients’ treatment experience on a scale unimaginable even a few years ago, Arabhao says.
“Our collaborator John Torous [Instructor of Psychiatry at Harvard Medical School] found our results reassuring, as these results reflect what he observed in his clinical experience,” Abrahao recalls. “At the same time, we need to complement our methodology with clinical patient data to link what we learn from social media to clinical implications.”
The researchers hope to validate their findings by contacting and interviewing many of the individual Twitter users whose posts they tracked.
Abrahao says he is confident that his group’s research have the potential to change best practices and regulations in psychiatric medicine.
“Sometimes doctors benefit from a second opinion. That opinion can come from an algorithm,” says Abrahao. “It shouldn’t replace their judgement, but AI-assisted medicine is something that could be used today.”